We are at the cusp of one of the largest technological inflections in our generation. Some industries will be uprooted and replaced; others will grow significantly as new markets are unlocked. With the proper framework, investors can be ready to take advantage of these inflections. For an introductory article on Generative AI, see our Part 1 here: https://pernasresearch.com/investment-writings/generative-ai/
LLMs “Think”
While much attention has focused on model training and discussions of “Stargate” data centers, inference workloads are poised to take center stage. About six months ago, LLMs faced criticism for “hallucinations” and their lack of Type 2 thinking—their inability to think critically and reliance on “one-shot” responses. With the recent ChatGPT Strawberry and o1 releases, however, a new picture is emerging.
This shift is due to increased inferencing compute. Rather than providing rapid, one-step responses, ChatGPT now employs a technique called “hidden chain of thought” reasoning, performing multiple internal steps to analyze the problem before responding. This has given rise to the second law of scaling, seen below. That is the more compute the LLM uses for inference, the more accurate the response ( the right graph2).
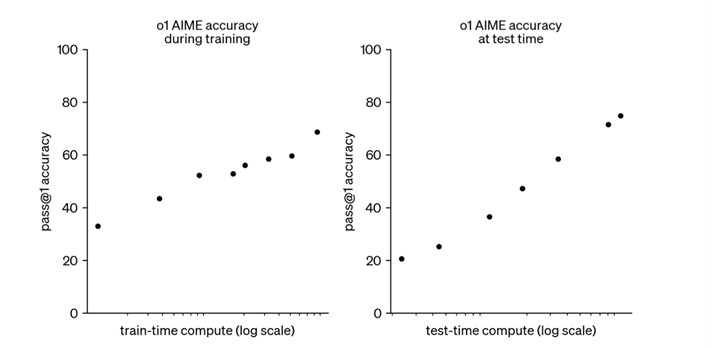
The rise of inference compute has been fueled by significant reductions in both cost and speed, thanks to specialized hardware and model optimization. For instance, NVLINK networking enables 72 Blackwell GPUs to function as a single unit, reducing “thoughtful inference time” to mere milliseconds. Hyperscalers like Amazon have also developed specialized AI hardware dedicated to inference.
The Rise of Inference and Implications
As inference workloads grow, they will reshape the dynamics between data centers and edge devices. In roughly five years, it’s likely that 80% of total compute will occur at the inference level, with only 20% dedicated to training. The trend of prolonged inference highlights the increasing role of edge data centers, which may soon surpass the importance of large hyperscale facilities.
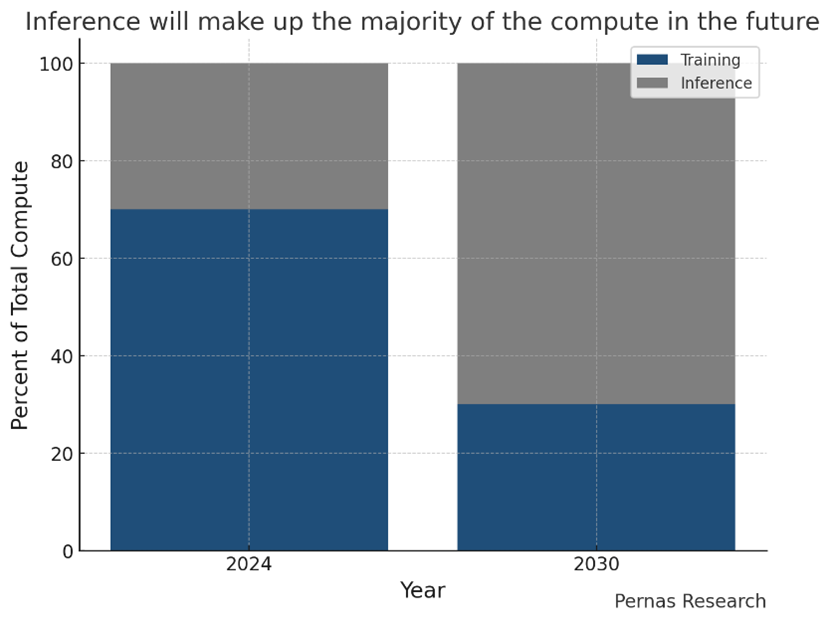
Edge data centers—typically smaller facilities with fewer than 50 racks located in urban areas—are a fragmented industry. However, with rising inference workloads, more computation may shift to the edge, potentially driving substantial upgrades. Edge data centers could see power consumption more than double as demand grows for real-time inference (e.g., generating movies from prompts like “Create Star Wars in the style of Christopher Nolan with a John Williams soundtrack”), increasing their importance.
Inference also places pressure on edge devices. Smartphones currently average around 8 GB of RAM, which would be fully consumed by even Small Language Models. By 2030, average RAM on smartphones and computers could exceed 50 GB. However, longer inference times allow for smaller, less data-intensive models, creating an equilibrium where specialized, compact models are tailored for edge devices.
Data & Agents
For investors, assessing how outdated a company’s tech stack is during a technological sea change has never been easier. However, predicting whether new technology will harm or benefit a company remains complex; while first-order effects may offer advantages, second-order effects can differ significantly. In the near term, the companies most likely to benefit from generative AI technology are those with 1) existing data advantages and 2) substantial headcounts in areas that can be streamlined through AI agents.
Is Data the New Oil?
Data is often touted by management teams as a competitive advantage, yet not all data offers the same value. For data to create a lasting advantage via AI, it should meet several key criteria: 1) uniqueness (not easily replicated or proxied), 2) volume (transformers improve with more data), 3) durability (data that retains value over time without strong recency effects), 4) quality (clean, unbiased data to avoid issues like model collapse), 5) integration (data aggregated from CRMs, ERPs, sensors, etc., then processed and cleansed), and 6) a positive feedback loop that iteratively improves models.
Although data currently provides a large advantage to incumbents, this advantage could depreciate significantly as the use of synthetic data increases. Synthetic Data is artificially generated data that mimics the real-world and is designed to have similar statistical properties. Synthetic Data is still plagued with many issues that compound as it’s usage propagates into more future models.
AI Agents
AI agents represent the potential energy of generative AI realized, shifting from knowledge repositories to action-oriented capabilities—a transformational leap. Soon, “swarms” of millions of agents could operate within companies, driving efficiencies, expanding revenues, and opening new markets. In the short term, however, companies will primarily leverage AI agents for cost reduction, leading to significant cost-curve disruption and a strong deflationary impact. Additionally, AI agents will accelerate an organization’s "clock speed," enhancing the pace of internal processes from sales to customer service. Companies focused on Business Process Automation, such as Salesforce and UiPath, are poised to benefit from this acceleration.
"I use Synopsys and Cadence for my Chip design, and I look forward to renting or leasing a million AI Chip Designer agents from Synopsys to design a new Chip" – Jensen Huang
While there are technical challenges in creating and implementing AI agents, the most significant hurdle will be the cultural shift required within companies. Organizational inertia and inherent biases often resist these changes. Roles most likely to be automated are those that involve high standardization and volume, such as customer service (e.g., call representatives), inside sales reps and sales development reps, where tasks like lead generation, answering questions, and order processing can be automated.
An example is “Alice”, shown below3, is a AI SDR Agent developed by 11x. Alice operates at 80% lower cost than human counterparts, isn’t constrained by geography or time zones, functions across all channels, and improves with each interaction.
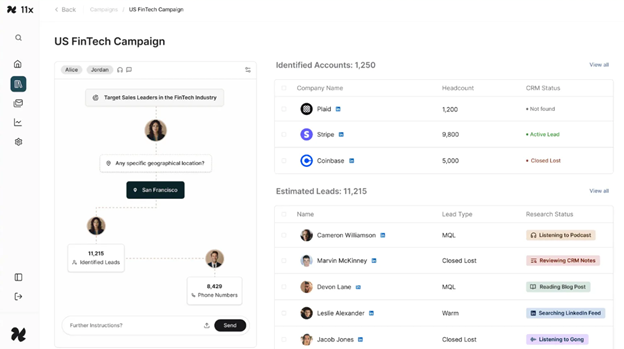
“I could see 100% of our SMB salesforce becoming AI owned..” Senior Director, ZenDesk
AI agents are also being developed for software engineering, enabling top programmers to perform the work of an entire team by assisting in code writing and evaluation. In financial services, AI agents are used for tasks like fraud detection and automated trading. For example, PayPal has effectively utilized AI to prevent fraudulent transactions, saving …