In this industry essay, we discuss:
- How data centers are evolving due to AI workloads and new hardware requirements
- The trends that will be occurring due to this evolution from compute to chiplet architecture
- The industry participants that are poised to benefit from this inflection
- Potential headwinds that could inhibit the adoption of this new data center architecture
The rise of AI
Traditionally, Data Centers have gone unnoticed, humming in the global background powering things such as Google's searches and Netflix streaming videos. However recently, data centers have taken the front seat due to Generative AI Revolution and advances in High Performance Computing. Jensen Huang, CEO and founder of Nvidia, forecasts that within the next four years, expenditures on data center equipment for AI workloads will reach an estimated $1 trillion.
With the tsunami of AI demand for compute and storage, the data center industry faces a pivotal challenge. The vast majority of existing data centers are structured around CPU-based servers, which are rapidly becoming inadequate for modern requirements. To address this, a significant overhaul is necessary to integrate the newer GPUs. Unlike CPUs, which process instructions sequentially, GPUs are capable of executing thousands of parallel computations simultaneously, offering a substantial computational edge and efficiency over the traditional, energy-intensive CPU servers. (Although H100 GPUs say are rated for a mind boggling 700 watts, they are much more efficient than CPUs). The new standard of performance is computation per watt, a critical factor in preventing the power requirements of data centers from becoming untenable. Despite the computational efficiency advancing exponentially, the demand for compute is increasing at an even greater rate. The appetite for AI-driven workloads, such as large language models (LLMs), is voracious, fueled by continuous innovation and applications, such as text-to-video generation. These models are expanding rapidly: for instance, Chat GPT 1 contained 1.5 billion parameters, whereas Chat GPT 4 now boasts 1.7 trillion. This shift from CPUs to GPUs necessitates a rearchitecture of data centers, spanning network infrastructure, cooling systems, and power management. We delve into what a data center is and hardware trends that will result from increasing AI adoption.
What is a data center?
A data center is a facility that houses many servers all interconnected via cabling and switches to enable data processing, storage, and distribution. A server is a personal computer on steroids: It possesses much greater RAM, computing power, and multiple redundancies. Data centers are the workhorse of every computational workload, they need to have extremely high fidelity and run times. Some data centers are only offline for 25 minutes in the entire year. Data centers are typically owned and operated either by co-location providers, telcos, or hyperscalers.
A data center has thousands of servers housed in racks. A rack is simply a cabinet full of servers. These servers range from computer servers to memory servers and connecting them is a switch. Traditional data center networks use a ‘spine and leaf’ configuration. In this configuration1, the leaf layer consists of switches that connect directly to terminal equipment such as servers, storage devices, and other networking components. Each leaf switch is interconnected with every spine switch, which facilitates communication between devices in the leaf layer and extends connectivity to other segments of the network via the spine layer. These layers are, in turn, connected to a backbone network, which links to routers that integrate the data center with the internet or other data centers. Approximately 80% of data transmission occurs internally within data centers.
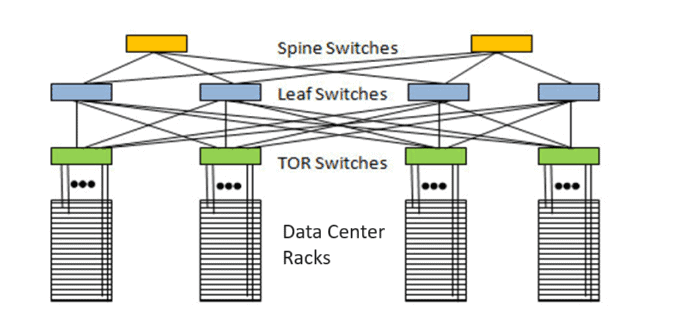
There are about 5,500 data centers in the USA, making up half the global number of data centers. The average data center holds about 100k servers and requires significant energy to run. Each rack typically consumes 15-20 kilowatts. Currently data centers in the USA make up about 2% of energy demand or about 80 TWhrs. A byproduct of this energy consumption is heat. Running data centers gives off tremendous heat and left unchecked, this would compromise the equipment, however with modern cooling methods, temperatures can be kept in a stable operating region. Surprisingly, about half the power consumed in a data center (shown below2) goes to cooling servers in order to have them be able to run for extended periods of time. The traditional methods of cooling are with air: Cool air enters the server through a fan, absorbs the heat from the heat sink and gets expelled out of the other side of the server.
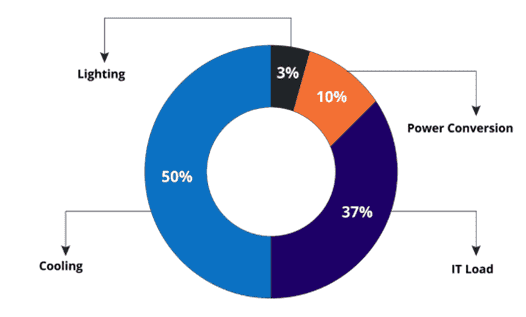
What’s next?
The traditional data center has not changed much since the 80s. That is until now. The latest data center rack unveiled at Nvidia's GTC 2024, "the Woodstock of AI": 72 Blackwell chips connected by 4 NVlink switches, liquid cooled, with 150 Tbps Infiniband (we go into more detail on these). It sounds like something out of a science fiction novel. With data centers becoming “AI Factories”, we believe that this is the first inning of major technological changes and delve into the data center trends below.
Trends
Network Debottlenecking
Jensen Huang recently debuted NVIDIA’s vision for ‘AI Factories’: These data centers on steroids contain about 32k GPUs with 645 exaFlops of compute. Compute has gone up 1000x in a decade and as long as there are returns to higher compute, demand will continue to increase. However, while GPUs have accelerated computation, other parts of the chain have not kept up: Namely data connectivity. This ranges from cabling, switches and specialized server chips to other components within the data center environment. Really anything that transmits data between servers and storage systems. Industry reports estimate that 30 percent of the time spent training a LLM due to network latency with the other 70 percent spent on compute. The very last bytes of data control when the next computation cycle begins, which means GPUs sit idle while data is being transmitted. This is compounded if data packets get dropped, called tail latency. These are the problems that beset just the training phase …